Combining computing and memory in a single unit with low-power electronics
MoS2-enabled logic-in-memory devices with the potential to outperform silicon
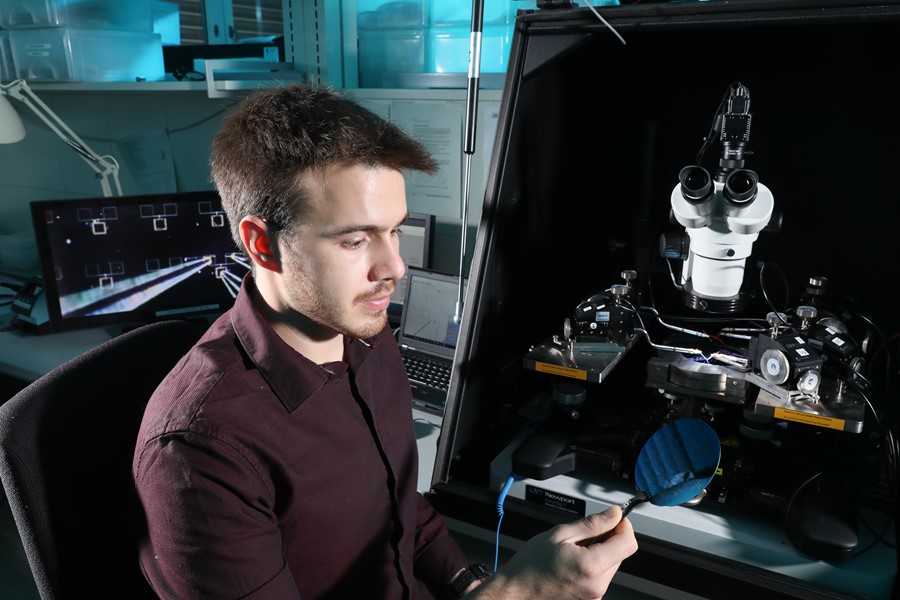
Guillherme Migliato Marega, the lead author on the two papers, inspecting a wafer with MoS2 circuits (Credit: Allain Herzog, EPFL)
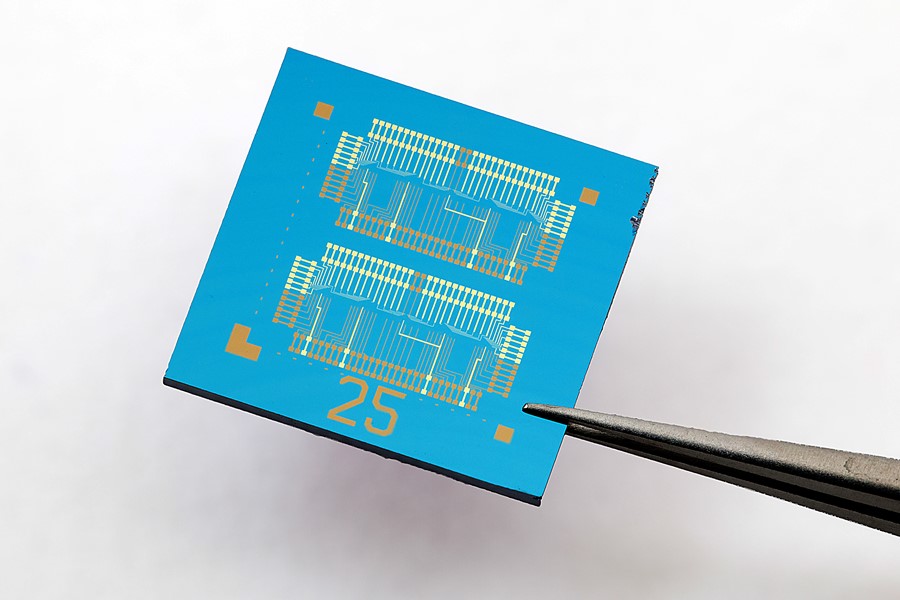
Chip based on floating-gate field-effect transistors (FGFETs) with an active molybdenum disulphide channel (Credit: Andras Kis)
Researchers from Graphene Flagship Partners École Polytechnique Fédérale de Lausanne (EPFL, Switzerland), and University of Pisa (Italy), in collaboration with University of Messina (Italy) developed a low-power brain-inspired model based on ultra-thin semiconducting molybdenum disulphide (MoS2). This includes circuits that behave like artificial neurons (perceptrons) and can potentially outperform their silicon counterparts.
The transfer of data between a computer’s processor and memory is responsible for over one-third of all energy used by our tech devices. This waste of time and energy is one of the limits of the current silicon-based computer architecture, where logic and memory reside in different components. Researchers are looking into new materials that could revolutionise our computers, by building processors that also work as memory storage devices. These systems, which mimic the workings of neurons, could speed up calculation-intensive applications, such as image processing, machine learning and artificial neural networks.
Expanding on prior research published in Nature in 2020, the Graphene Flagship team devised a processor which includes an array of floating-gate memories – devices capable of trapping electrons for long periods – using monolayer MoS2 as the active channel. Floating gate transistors are already present in flash memory systems, used in cameras, smartphones and computers. The new transistors based on MoS2 can be made much smaller and consume less energy. Being so thin, they are also more sensitive than silicon to differences in the number of electrons coming in and out of the floating gate. By adding or removing electrons from the floating gate, the Graphene Flagship team achieved 16 different levels of conductivity in the MoS2 channel. This enables access to greater possibilities compared to silicon-based memory devices, where it is difficult to program more than two levels: conductive or non-conductive.
The researchers assessed the device’s performance in recognising numbers on liquid crystal displays (LCDs), like digits in digital watches, and obtained an experimental accuracy of up to 91.5%. To do this, the team used a so-called artificial neural network, a circuit which resembles a cluster of neurons connected to each other. The ability of MoS2 memories to store different levels of electrical conductivity was used to mimic the strength of connections between neurons.
“We went beyond computer-simulation and produced a working circuit with high quality ultra-thin materials,” says Andras Kis from Graphene Flagship Partner EPFL, who led the study.
Recognising these digital numbers can be considered one of the simplest forms of AI-based image recognition. The Graphene Flagship team also showed that, if these circuits were upgraded and trained to recognise specific objects in regular photos, they could consume almost 40 times less power than state-of-the-art silicon-based circuits.
This processor was built with memory devices only 180 nanometres long and the team claims that they could shrink them down to 50 nanometres without significant loss of performance. Here again this MoS2-enabled device could beat silicon: reducing the size of silicon memory devices is difficult because electrons tend to leak out from the floating gate.
“Layered materials, especially the polycrystalline or amorphous forms of BN and transition metal dichalcogenides, are now being seriously considered for developing innovative memristive devices, with potential impact on low-energy information storage and processing. This work is a new milestone for the fabrication of energy-efficient devices, mimicking artificial neurons, outperforming competing silicon devices and paving the way towards future neuromorphic computing architectures,” says Stephan Roche, Graphene Flagship Leader of the Division Enabling Science & Materials.
Andrea C. Ferrari, Science and Technology Officer of the Graphene Flagship, adds: “Low energy consumption neuromorphic computing devices are centre stage in the Graphene Flagship activities focussing on electronics based on graphene and related materials. This work creates a strong foundation on which to build future efforts to translate these results into innovative devices, and for the Horizon Europe phase of the Graphene Flagship”.
References
Migliato Marega, Guilherme, et al. "Low-Power Artificial Neural Network Perceptron Based on Monolayer MoS2." ACS Nano (2022). https://pubs.acs.org/doi/abs/10.1021/acsnano.1c07065
Marega, Guilherme Migliato, et al. "Logic-in-memory based on an atomically thin semiconductor." Nature 587.7832 (2020): 72-77. https://www.nature.com/articles/s41586-020-2861-0